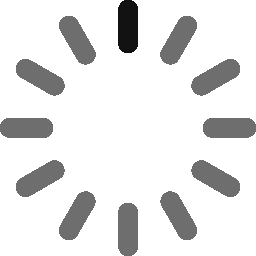
Related items loading ...
Section 1: Publication
Publication Type
Journal Article
Authorship
Gauch, M., & Lin, J.
Title
A Data Scientist's Guide to Streamflow Prediction
Year
2020
Publication Outlet
arXiv preprint arXiv:2006.12975
DOI
ISBN
ISSN
Citation
Gauch, M., & Lin, J. (2020). A Data Scientist's Guide to Streamflow Prediction. arXiv preprint arXiv:2006.12975.
Abstract
In recent years, the paradigms of data-driven science have become essential com-
ponents of physical sciences, particularly in geophysical disciplines such as clima-
tology. The field of hydrology is one of these disciplines where machine learning
and data-driven models have attracted significant attention. This offers significant
potential for data scientists’ contributions to hydrologic research. As in every
interdisciplinary research effort, an initial mutual understanding of the domain is
key to successful work later on. In this work, we focus on the element of hydrologic
rainfall–runoff models and their application to forecast floods and predict stream-
flow, the volume of water flowing in a river. This guide aims to help interested data
scientists gain an understanding of the problem, the hydrologic concepts involved,
and the details that come up along the way. We have captured lessons that we have
learned while “coming up to speed” on streamflow prediction and hope that our
experiences will be useful to the community.
Plain Language Summary