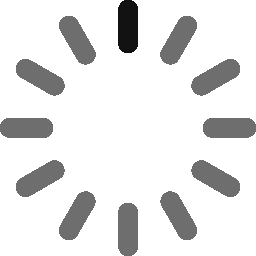
Related items loading ...
Section 1: Publication
Publication Type
Journal Article
Authorship
Kowshal, A., Das, A., & Lindenschmidt, K. E.
Title
Developing a geospatial model to predict locations of higher propensity to ice jamming along the Saint John River
Year
2021
Publication Outlet
CGU HS Committee on River Ice Processes and the Environment, 21st Workshop on the Hydraulics of Ice Covered Rivers Saskatoon, Saskatchewan, Canada, August 29 - September 01, 2021
DOI
No DOI Available
ISBN
ISSN
Citation
Kowshal, A., Das, A., & Lindenschmidt, K. E. (2021). Developing a geospatial model to predict locations of higher propensity to ice jamming along the Saint John River. CGU HS Committee on River Ice Processes and the Environment, 21st Workshop on the Hydraulics of Ice Covered Rivers Saskatoon, Saskatchewan, Canada, August 29 - September 01, 2021.
Abstract
During spring breakup, ice-jam related flooding is a dangerous threat to many
riverine communities in cold regions. Ice-jam floods can be relatively severe and
often more damaging than open-water floods since they often occur suddenly and
are associated with much faster and higher staging. Moreover, various
geomorphological characteristics can be responsible for severe ice-jam
formation. Therefore, it is necessary to identify the propensity of a river channel
to ice-jam formation in order to develop an effective flood mitigation strategy
that could reduce potential flood damages. Although several geospatial models
have already been developed for various rivers across Canada, a geospatial model
precisely focused on the Saint John River das not been developed. The main
purpose of this work is to introduce an advanced Machine Learning Approach
(MLA) that has been used to develop a geospatial model based on
geomorphological parameters (e.g. sinuosity, slopes and depths) and features
(e.g. islands or sandbars, narrow channels, tributaries, bridge piers). A hydraulic
model (RIVICE) was used to simulate both solid ice cover slopes and longitudinal
profiles of riverbed elevations (depths). The remaining geomorphological
parameters were derived from spatial data using a Geographical Information
System (GIS) tool. Based on observed ice-jam data, a data-driven machine
learning model was applied to the aforementioned factors to cluster
geomorphological parameters. First, the trained model was used to identify the
most probable ice-jam locations and then the results were applied in the test
model domain to determine the accuracy of the model
Plain Language Summary
Section 2: Additional Information
Program Affiliations
Project Affiliations
Submitters
Publication Stage
Published
Theme
Presentation Format
Additional Information
IMPC, Refereed Publications