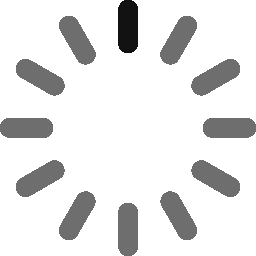
Related items loading ...
Section 1: Publication
Publication Type
Journal Article
Authorship
Gauch, M., Mai, J., Gharari, S., & Lin, J.
Title
Data-driven vs. physically-based streamflow prediction models
Year
2019
Publication Outlet
In Proceedings of 9th International Workshop on Climate Informatics
DOI
ISBN
ISSN
Citation
Abstract
Climate change leads to more frequent and severe floods and droughts. Precise water flow forecasts for rivers and streams help mitigate damage and are direly needed. We evaluate physically-based and data- driven models on the task of streamflow prediction in the Lake Erie region: Physically-based models capture simplified representations of the physical processes that underlie streamflow, while purely data-driven models encode no such knowledge explicitly. Experiments show that data-driven approaches can provide more accurate predictions than a physically-based model, suggesting potential in hybrid approaches that combine hydrological understanding with high prediction accuracy.
Plain Language Summary