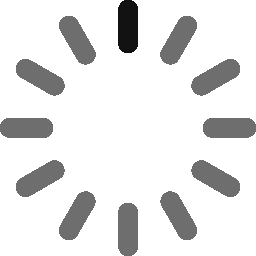
Related items loading ...
Section 1: Publication
Publication Type
Conference Proceeding
Authorship
Bryan, T., Mai, J., & the GRIP-E/GL Project Team
Title
The Runoff Model-Intercomparison Project over Lake Erie and the Great Lakes
Year
2020
Publication Outlet
In EGU General Assembly, May 2020
DOI
ISBN
ISSN
Citation
Bryan, T., Mai, J., & the GRIP-E/GL Project Team (2020). The Runoff Model-Intercomparison Project over Lake Erie and the Great Lakes. In EGU General Assembly, May 2020.
https://doi.org/10.5194/egusphere-egu2020-10319 Conference Proceeding
Abstract
The Great Lakes Runoff Inter-comparison Project (GRIP) includes a wide range of lumped and distributed models that are used operationally and/or for research purposes across Canada and the United States. Participating models are GEM-Hydro, WRF-Hydro, MESH, VIC, WATFLOOD, SWAT, mHM, Noah-MP, HYPE, LBRM, GR4J, HMETS, and purely statistical models. The latter are added to assess the information content of the forcing and geophysical datasets. As part of the Integrated Modelling Program for Canada (IMPC) under the Global Water Futures (GWF) program, the project is aiming to run all these models over several regions in Canada. We started with the Lake Erie watershed and then extended the study to the whole Great Lakes domain.
One of the main contributions of the project is that we identified a standard dataset for model building that all participants in the inter-comparison project can access and then process to generate their model-specific required inputs. The common dataset allows identifying differences in model outputs that are solely due to the models and not the data used to setup the models. This presentation will give an update on the design of the inter-comparison and will report on comparative results for two sets of streamflow gauging stations: A) gauge stations with low-human impact upstream watersheds and B) most down-stream gauge stations directly draining into the lake(s).
The main results are: 1) The best performing semi-distributed model calibrated across all stations at once is HYPE. The mHM is the best distributed model calibrated at each station individually (median NSE = 0.78) while LBRM is the lumped model that is on average the best (median NSE = 0.66). 2) The purely statistical model is highly competitive with and even slightly outperforming all hydrologic models except mHM in the calibration period. 3) The performance of most models decreases in urbanized areas. Only models that are calibrated independently at each station are capable of modelling urbanized areas. 4) No significant change in performance can be observed between low-human impact watersheds and watersheds that are mostly downstream, draining directly into a Great Lake.
Plain Language Summary