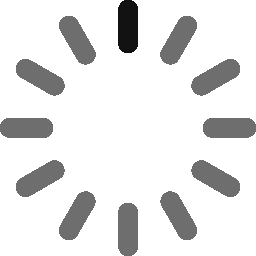
Related items loading ...
Section 1: Publication
Publication Type
Conference Presentation
Authorship
Casson, D., Knoben, W., Arnal, L., Gharari, S., van Osnabrugge, B., Tang, G., Liu, H., Clark, M.
Title
Ensemble Data Assimilation Methods for Improved Snow Estimation and Streamflow Prediction in Mountainous Terrain
Year
2022
Publication Outlet
IAHS-AISH Scientific Assembly 2022, Montpellier, France, 29 May–3 Jun 2022, IAHS2022-572
DOI
ISBN
ISSN
Citation
Casson, D., Knoben, W., Arnal, L., Gharari, S., van Osnabrugge, B., Tang, G., Liu, H., Clark, M. (2022) Ensemble Data Assimilation Methods for Improved Snow Estimation and Streamflow Prediction in Mountainous Terrain, IAHS-AISH Scientific Assembly 2022, Montpellier, France, 29 May–3 Jun 2022, IAHS2022-572
Abstract
Accurate estimation of seasonal snow mass for streamflow forecasting remains a technical and scientific challenge that requires advances in both physically based modelling and measurement techniques. Data assimilation provides methods to optimally combine modeled and measured information, and can be used to improving snow state estimates used as initial conditions for streamflow forecasting. Several key challenges remain for practical implementation in mountainous snow data assimilation, including quantification of measurement and model uncertainties, connecting point-scale observations to spatially distributed model states in complex terrain and the ability to improve information where measurements are not available.
This research presents recent effort in addressing these challenges through ensemble snow data assimilation in the Canadian Rocky Mountains. Specifically, discretization to improve spatial representation of snow cover, assimilation of in-situ measurements with the Particle Filter and Ensemble Kalman Filter and assessment of the impact on streamflow forecasts. This is carried out with a dynamic multi-layer, energy balance snow model in the Structure for Unifying Multiple Modeling Alternatives (SUMMA) framework. This builds on recently developed North American domain hydrological modelling, probabilistic meteorological data generation and forecasting efforts by the Computational Hydrology group at the University of Saskatchewan. Planning for snow sub-grid heterogeneity and the assimilation of remotely sensed fractional snow cover area will also be presented.
Plain Language Summary